Optical quality assurance through automated image recognition
In every production process, various influences result in products that no longer meet the quality requirements. There are various methods for sorting these out. The oldest method is visual inspection by employees.
Thanks to their highly developed and extremely improvisational brains, humans are able to transfer a comprehensive and complete understanding of quality requirements to the product and, in the event of a deviation, intuitively decide correctly between "flawless" and rejects.
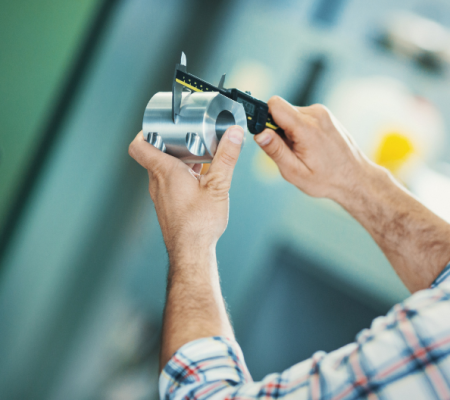
Manual quality inspection of components
Automated visual inspection in its infancy
In the course of automation due to cost pressure and competitiveness in the continuously opening international market, machines are increasingly being used in quality control. Due to the frequently existing threshold and limit values in the quality specifications and the usually necessary traceability of decisions, rule-based computer vision systems have become established. Manufacturers of such technologies have created very powerful methods that often outperform humans in terms of speed and measurement accuracy. However, these systems require extremely homogeneous conditions in terms of lighting, working distance, camera perspective, object background, etc. The objects to be inspected and their surfaces must also meet a high degree of homogeneity. Therefore, these machines are often equipped with appropriate chambers within which homogeneity is guaranteed, making the systems very large and expensive. Furthermore, complex decision trees often have to be created for each product to be tested. In summary, these procedures can be described as complex, rather rigid expert systems that are tailored to a very specific case, but within which they can operate very efficiently.
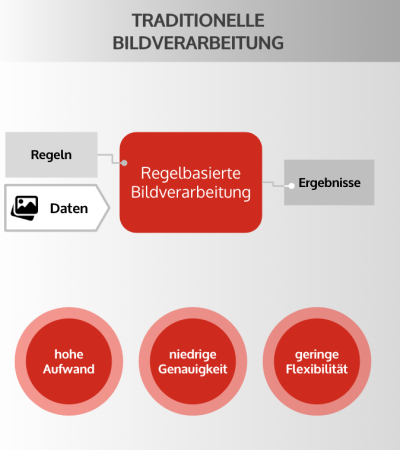
Computer vision process image
Automated visual inspection 2.0
The main reason for the necessary homogeneity and the "island talent" of these systems lies in their rule-based approach. For example, a crack could be introduced into the system as a dark, elongated shade using rules. By changing the lighting, however, it is possible that the crack is not a shade but a brightening and therefore may no longer be reliably recognized if this was not taken into account in the rules.
Humans, on the other hand, internalize their understanding of a crack mainly on the basis of examples, "supervised learning" in the AI sense, and their enormous basic knowledge of natural conditions such as the change of shadow and reflection in changing lighting conditions. Humans have a general understanding of a crack or blowhole in a cast object and can therefore perceive it as such based on a few individual sample images despite different lighting, color, viewing angle, background, etc. The principle of conceptual, supervised learning based on examples is also the basis of machine learning, which is supplemented by automated, hierarchical feature extraction of deep neural networks, also known collectively as deep learning. Of course, an artificial neural network, no matter how complex, does not have the same prior knowledge that a human brings to the table, but has to learn this based on many example images relating to a specific case. But here too, the use of pre-trained networks significantly reduces the amount of sample data, as the network has already learned basic concepts such as edges, shading or even cracks or scratches in a different context. The concept behind this is known as transfer learning and is actually an analogy to the learning concepts in the brains of many living creatures.
With the help of deep learning as a bridge between the natural, intuitive understanding of human concepts and automated evaluation, the essential advantages of both worlds can be utilized. elunic is already using this technology very successfully in many applications with AI.SEE™:
You might also be interested in
More machine learning services from elunic
Get in touch.
Do you have a project enquiry? Please do not hesitate to contact us - we will be happy to answer you promptly.
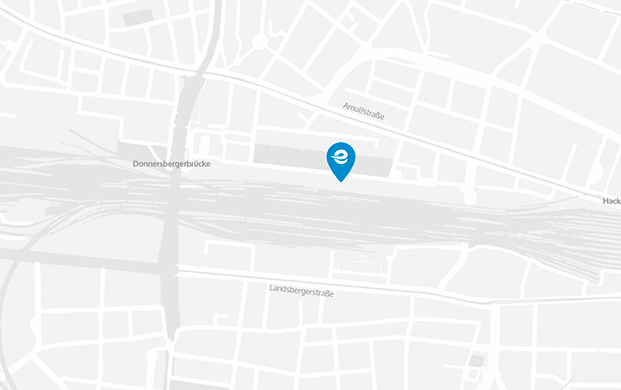