Predictive maintenance in Industry 4.0
Predictive Maintenance:
AI agent-driven progress in Industry 4.0
Predictive maintenance is more than just a trend - it is a revolution in Industry 4.0, fuelled by AI agents. These intelligent systems enable machine operators to not only prevent breakdowns and downtimes of their systems, but to strategically predict and control them.
The use of AI agents in real-time data monitoring takes predictive maintenance to a new level. Sensors and monitoring systems collect machine data that is analyzed by AI agents. These intelligent systems use advanced algorithms such as machine learning (ML) and artificial intelligence (AI) to make precise predictions about the condition of the machines. They identify anomalies and potential problems at an early stage, allowing preventive maintenance measures to be initiated in a targeted manner.
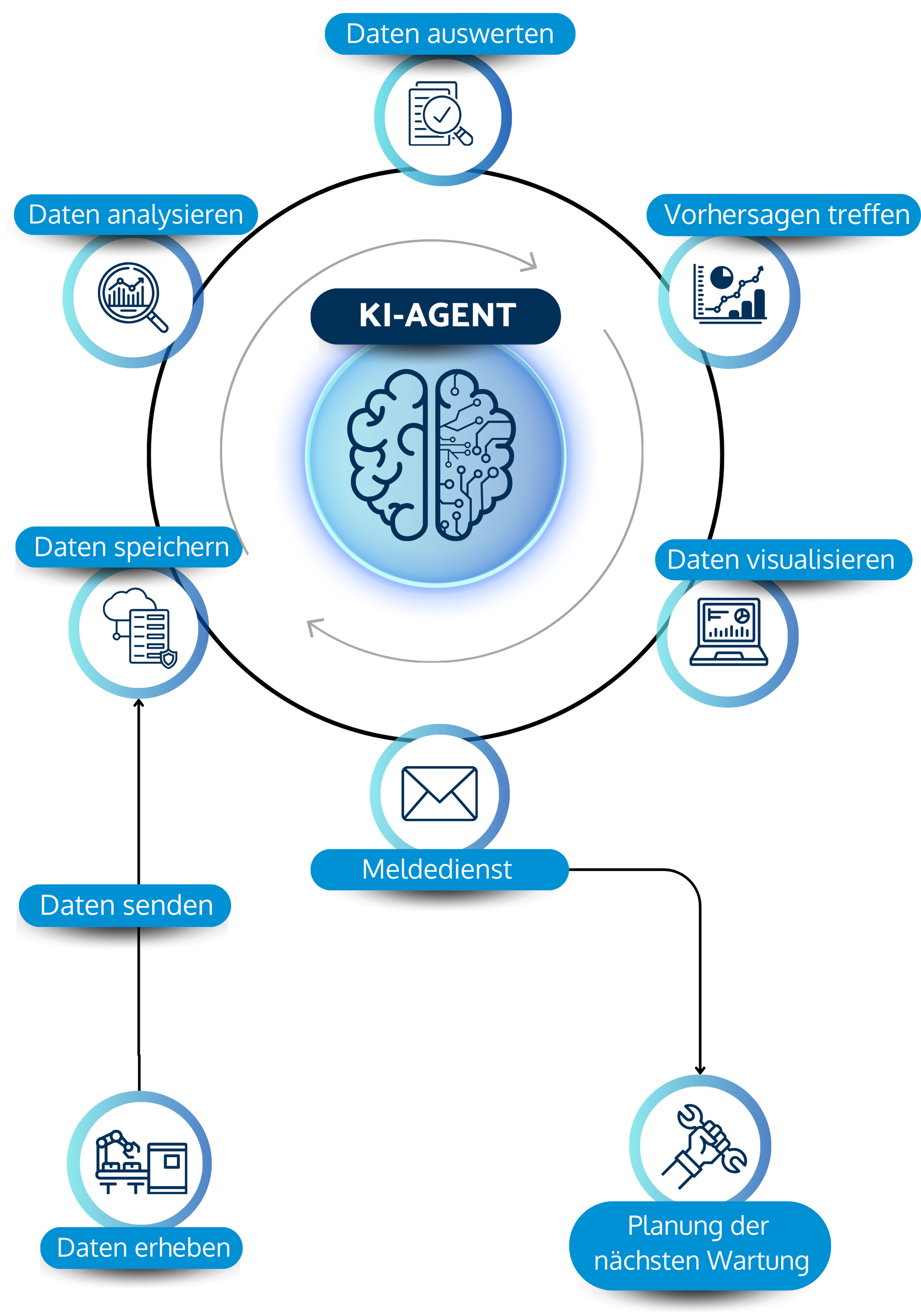
The advantages of AI-controlled predictive maintenance:

elunic AG: Your service provider
for Predictive Maintenance
Accelerate digitalisation significantly.
6 steps to a successful predictive maintenance project
Frequently asked questions
- When is predictive maintenance worthwhile?
Predictive maintenance is worthwhile if you have critical systems, want to reduce high maintenance costs, have access to relevant data and want to extend the service life of your systems. It can also be useful in safety-critical industries and for creating competitive advantages. A precise cost-benefit analysis is advisable.
- How is data collected for predictive maintenance?
- What is the difference between predictive maintenance and preventative maintenance?
Predictive maintenance uses data analytics and sensors to predict maintenance needs, while preventative maintenance is based on predetermined schedules to perform preventative maintenance, regardless of the current condition of the equipment. Predictive maintenance is data-driven and can be more cost-efficient.
- How is AI used in predictive maintenance?
In predictive maintenance, AI agents are used to optimise the maintenance and monitoring of industrial systems. This is mainly done through three key processes:
- Data acquisition and processingAI agents continuously collect data from various sensors and systems that indicate the physical and operational conditions of the machines. This includes vibration data, temperature measurements, energy consumption and other operating parameters. This data is pre-processed to reduce noise and extract relevant features.
- Pattern recognition and modellingAI uses machine learning (ML) techniques, in particular supervised and unsupervised learning methods, to recognise patterns from this data. Using historical data in which known machine failures and their signs are documented, the AI agent trains models to evaluate the current state of a machine. Using methods such as regression, classification or anomaly detection, the AI agent learns to identify early warning signals for potential failures or maintenance requirements.
- Forecasting and decision-makingBased on the recognised patterns and the trained model, the AI agent can make predictions about the future condition and maintenance requirements of the machines. These predictions enable the maintenance teams to act proactively instead of reacting to failures. The AI can also provide recommendations for optimal maintenance intervals and procedures to maximise operational efficiency and minimise downtime risks.
In addition, advanced AI systems in predictive maintenance often also integrate feedback loops in which the results of the maintenance measures carried out contribute to the continuous improvement of the prediction models. This leads to constant optimisation and adaptation to changing operating conditions and machine states.
Successful AI projects with shopfloor.io
Get in touch.
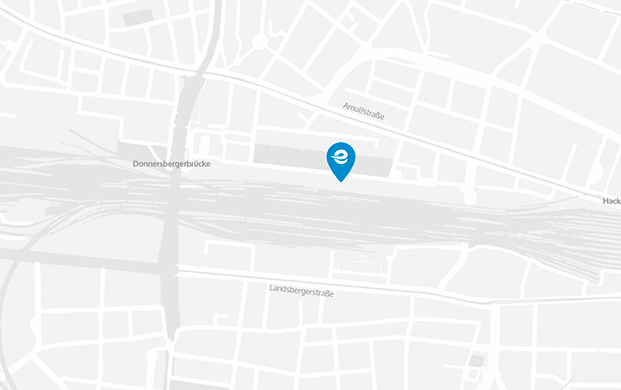