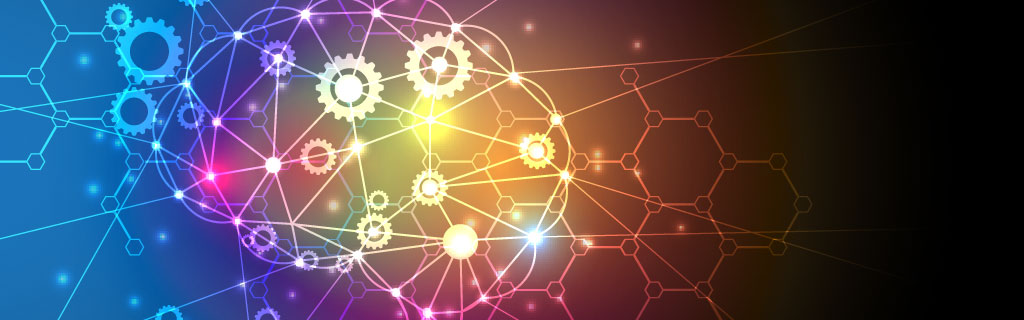
Utilise the benefits of deep learning in quality control with AI.SEE™
Ever increasing Quality requirements with simultaneous cost sensitivity make a Efficient quality control in the majority of the Industry indispensable.
Reliable and immediate detection of production faults can not only lead to a significant Lowering additional Costs for subsequent improvements, but also the financial loss in the event of Committee clear reduce or even prevent them. In addition, well-implemented detection of defects in production contributes to significant cost savings. quality- and Increased efficiency in the process. In most cases, an automated solution based on artificial intelligence proves to be optimal. AI.SEE™ from elunic impressively demonstrates the Advantage from Deep learning compared to classical methods of Machine learning.
Information processing without complicated formulas
Deep learning refers to a special method of Information processing. As a sub-area of machine learning, this uses "multilayer Learning", artificial neural networks with numerous intermediate layers (so-called hidden layers) to form a comprehensive internal structure between the input and output layers. This neuronal Structure enables a computer-based solution to learn from sample data in order to solve tasks that are difficult to formulate manually in mathematical rules. While tasks such as speech or facial recognition, which a person solves intuitively, seemed difficult for computers to solve until a few years ago, deep learning makes this possible today. As raw sensory input data, such as handwriting, can be recognised by software using such methods of information processing, deep learning offers almost unimaginable possibilities for the development of new technologies. Industry. This is largely possible without extensive expert knowledge to convert the countless pixels into a chain of numbers and letters. Rather various Techniques like Folding and Maximum selection used to analyse the essential Information on from the huge amounts of data Extract.
The way this computer-based learning works is inspired in many areas by learning in the human brain. The machine is thus enabled to self-employed and without large Involvement to continuously improve their abilities by using existing data and information to Sample extracted and Classified. The insights gained from this can then be correlated with data and linked in an extended context. This creates ever deeper and deeper layers of links that enable the machine to make decisions based on these links. The more correlations that arise from the data over time, the more complex issues can be mapped. Deep learning is therefore suitable for Applications The neural network is particularly suitable for applications where large amounts of data are available for deriving patterns and models. In addition, it is also suitable for applications that are able to form further correlations and thus an ever deeper understanding of the facts for the neural network over the course of time through ever new and larger data sets. Deep learning is therefore a good Basis for a automated visual Quality control in production.
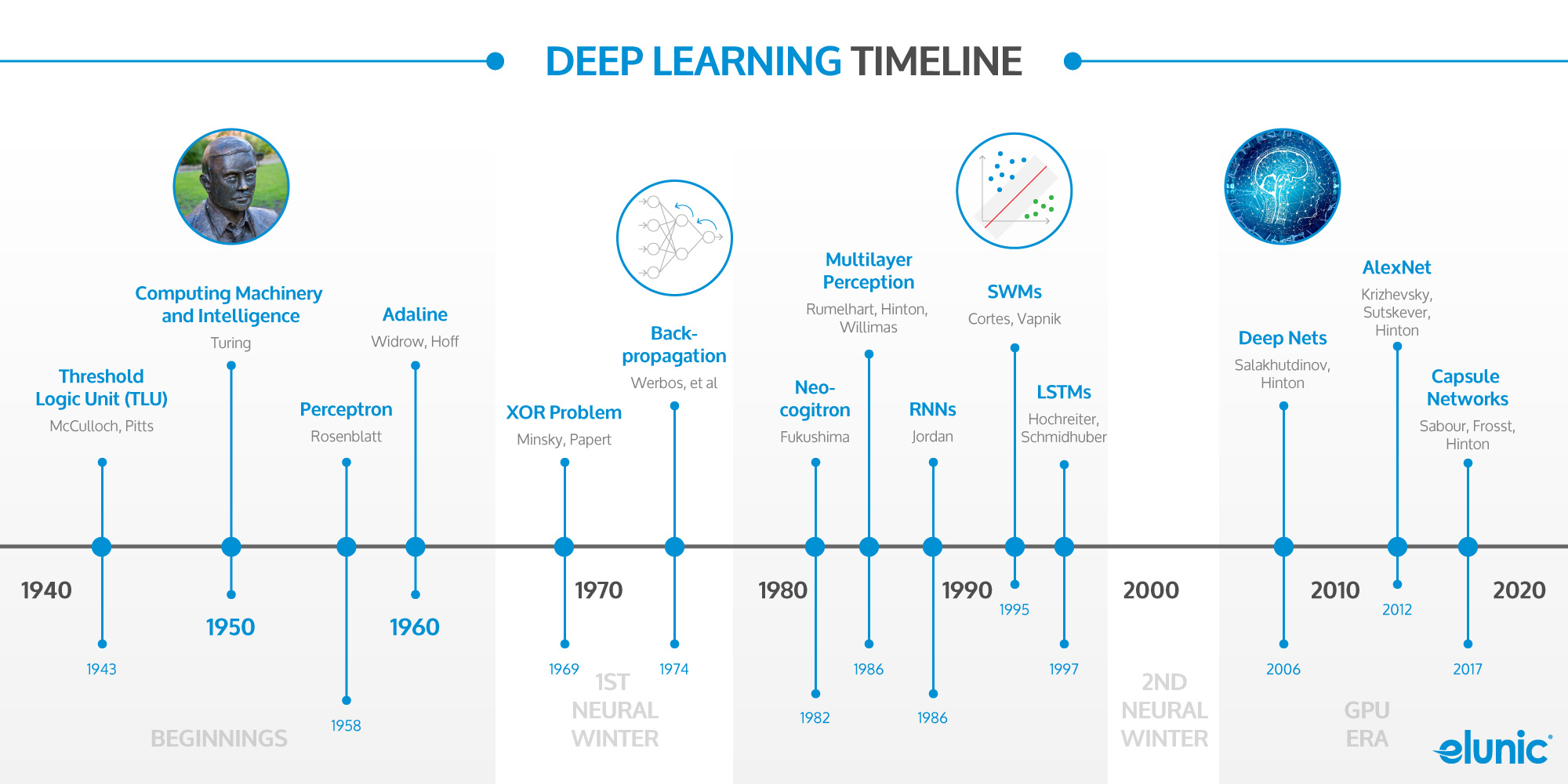
Deep Learning Timeline
Deep learning vs. "classic" machine learning in industry - the advantages at a glance
Although deep learning is a Subarea machine learning, it can be easily differentiated from "classic" machine learning. Methods of the "classic" Machine Learning are characterised by the fact that they make it possible to learn the relevant correlations for differentiation or prediction from the essential features. However, human expert knowledge and skills are still required to extract the feature data. The main advantage of deep learning is that this step, known as feature extraction, is also essentially automated.
The role of humans in the overall process therefore changes through the use of deep learning. Their task is to Information, mostly in the form of image data, for learning to provide. He leaves the analysis and derivation of a forecast or decision to the system itself. The relevant decision patterns are derived from the input data (images) provided to the software. extracted and the basic relationships in relation to the output (e.g. error class assignment) within the training process learnt. Thus, the main difference between "classic" machine learning and deep learning lies in the Capability, also unstructured data to process. This fact describes the greatest advantage of deep learning for visual quality control.
In this way, systems for quality control when using deep learning methods can be Patterns in the data, such as the shape, size and depth of the shadow cast by a Cracks and also recognise them. The result is a constantly optimising system that can also deal with rare production errors in the industry. highest Efficiency can recognise.
AI.SEE™ - using deep learning to achieve more effective quality control
With the automated Quality control AI.SEE™ elunic offers an application that is individually specialised for the respective production process, which Fully automated optical analyse and for all types of defects, such as casting defects, Blowhole oder Risse prüfen kann. Durch ihre einfache und äußerst intuitive Handhabung stellt AI.SEE™ die optimale Lösung zur visuellen Qualitätsprüfung jeder Art dar, die mit Hilfe von künstlicher Intelligenz, Produktionsfehler zuverlässig erkennt und dokumentiert. Eine umfangreiche Fütterung mit unmissverständlichen Trainingsdaten ermöglicht es, anhand verschiedener Fehlerbilder und Ausschusskriterien ein adaptiv lernfähiges Modell auf Basis von Methoden des Deep Learnings zu erschaffen und in der Lage ist, auch unscheinbare oder sich ändernde Fehlerbilder zuverlässig zu erkennen. Zusätzliche Fehlerbilder in der laufenden Produktion oder neue Produktreihen können in wenigen Schritten in das System integriert und so von diesem erlernt werden. Eine einfache and intuitive Bedienbarkeit der Anwendung stellt hierbei sicher, dass jeder Mitarbeiter in der Qualitätssicherung die Anwendung ohne Programmieraufwand mit Trainingsdaten füttern kann. Der offene Zugang zum System eröffnet zudem Möglichkeiten für weitere Unternehmensbereiche oder auch externe Dienstleister. So sind auch diese in der Lage, Modelle or Pipelines zu entwickeln und in das System zu integrieren.
Eine umfangreiche Datensammlung und –analyse der cloudbasierten Software ermöglicht zudem eine umfassende Rückverfolgung fehleranfälliger Bauteile und deren Überarbeitung im jeweiligen Produktionsschritt. Neben den Maßnahmen zur Optimierung zukünftiger Produktionschargen bietet die Implementierung einer Real time–Überwachung außerdem zahlreiche Möglichkeiten, um Ausschusszahlen zu senken.
Interesse geweckt? Dann informieren Sie sich jetzt über die zahlreichen Möglichkeiten der Qualitätssicherung durch AI.SEE™! Oder testen Sie direkt ob Ihre Probleme mit Deep Learning lösbar sind: